All our courses take place on-site at the University of St.Gallen.
Additionally, we are offering the following 6 courses in a HYBRID format - you can participate either online or on-site:
- Case Study Methods
- Machine Learning with R - Introduction
- Machine Learning with R - Advanced
- Mediation, Moderation, and Conditional Process Analysis I
- Qualitative Bayesian Reasoning for Case Studies
- Data Scraping and Management for Social Scientists with R
More information can be found in the course descriptions.
Register until 30 April!
- Case Study Methods
- Machine Learning with R - Introduction
- Machine Learning with R - Advanced
- Mediation, Moderation, and Conditional Process Analysis I
- Qualitative Bayesian Reasoning for Case Studies
- Data Scraping and Management for Social Scientists with R
More information can be found in the course descriptions.
Register until 30 April!
Boost your analytical skills
The Global School in Empirical Research Methods (GSERM) is a sophisticated programme on qualitative and quantitative methods launched by the University of St.Gallen.
Boost your analytical skills
The Global School in Empirical Research Methods (GSERM) is a sophisticated programme on qualitative and quantitative methods launched by the University of St.Gallen.
All our courses take place on-site at the University of St.Gallen.
Additionally, we are offering the following 6 courses in a HYBRID format - you can participate either online or on-site:
- Case Study Methods
- Machine Learning with R - Introduction
- Machine Learning with R - Advanced
- Mediation, Moderation, and Conditional Process Analysis I
- Qualitative Bayesian Reasoning for Case Studies
- Data Scraping and Management for Social Scientists with R
More information can be found in the course descriptions.
Register until 30 April!
- Case Study Methods
- Machine Learning with R - Introduction
- Machine Learning with R - Advanced
- Mediation, Moderation, and Conditional Process Analysis I
- Qualitative Bayesian Reasoning for Case Studies
- Data Scraping and Management for Social Scientists with R
More information can be found in the course descriptions.
Register until 30 April!
Why the course is worthwhile
Apart from participating a high-calibre integrated generic programme you can earn ECTS or enhance your career while learning more about qualitative and quantitative methods.
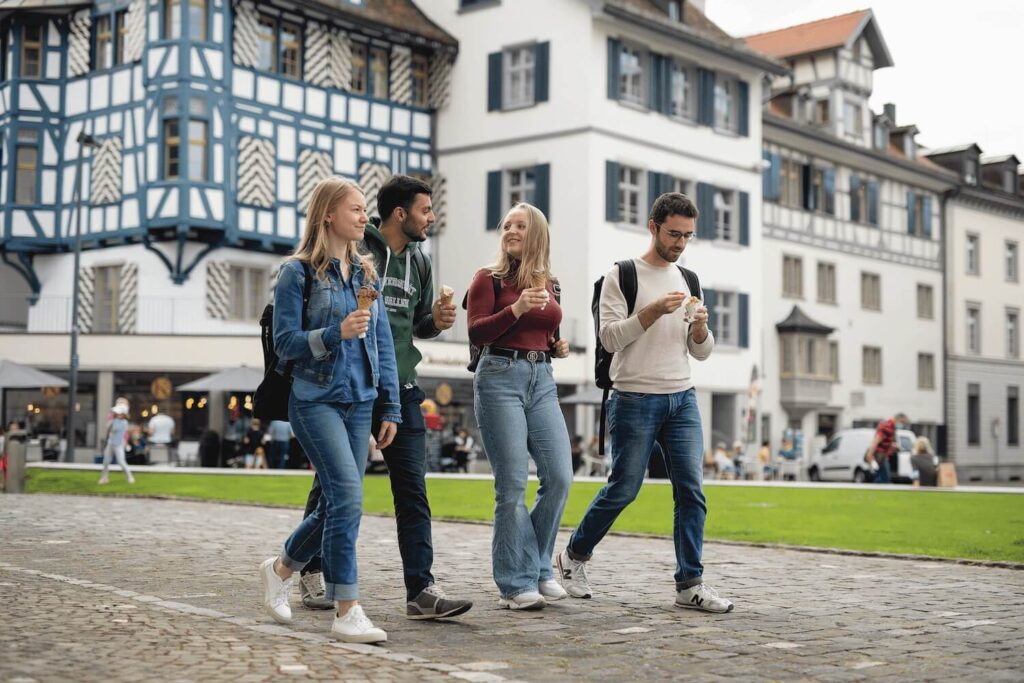
01
Networking with fellow researchers.
We not only welcome master and PhD students, postdocs, and graduate students from all kinds of study fields, but also professionals working outside of academia.
02
Compact block seminars.
You enhance your skills in block seminars taught by world-class faculty amongst an international crowd of participants. Participants choose one course per week (24 contact hours) worth 4 ECTS.
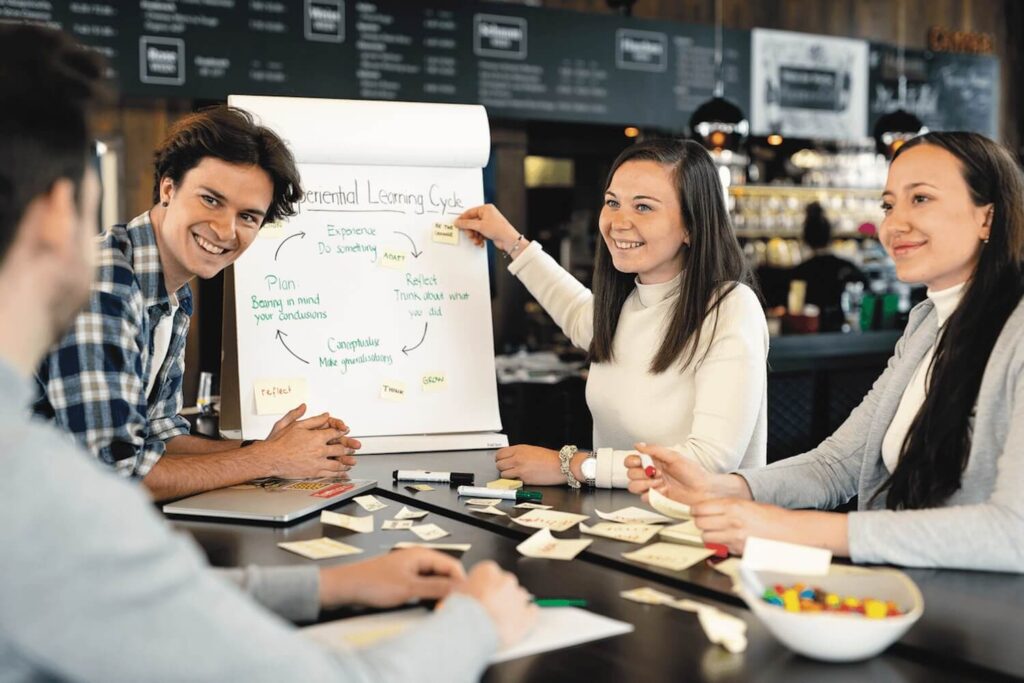
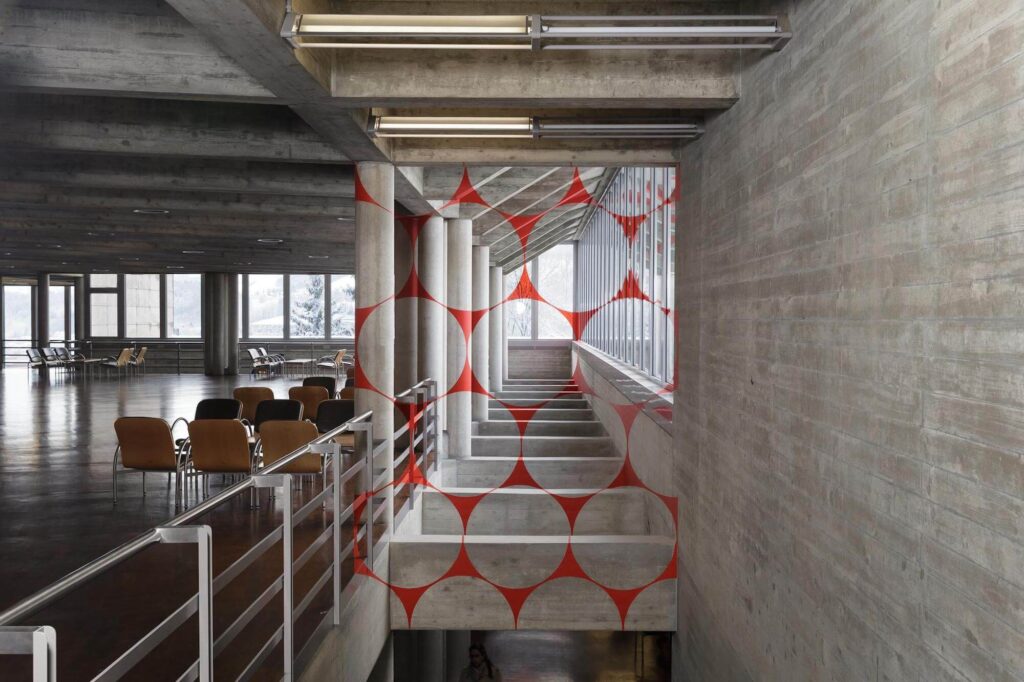
03
Interesting cultural programme.
At different locations in Europe we offer courses in quantitative and qualitative statistics and participants enjoy an interesting cultural programme.
Course benefits
You benefit a great deal while taking part on our courses.
1
Certificate of Attendance
Certificate of Attendance
Digital Certificate of Attendance will be issued if you attended at least 80 % of the course
2
Transcript with
4 ECTS
Transcript with
4 ECTS
University of St. Gallen transcript with 4 ECTS if you successfully pass the exam. Taking the exam is not mandatory.
3
Networking with international peers
Networking with international peers
Network with fellow researchers from all over the globe and very devoted international GSERM faculty
4
World-class faculty
World-class faculty
Knowledge transfer from a world-class faculty.
Testimonials
GSERM receives regularly excellent feedbacks and comments from our lecturers and participants and likes to share a selection of theses testimonials with you.
"I really enjoyed the GSERM Winter School. My week at Oslo was a good balance of academic learning and social networking organized in a smart and effective manner. In addition to learning from well published scholars and interacting with peers from all over the world, I was able to experience the Nordic way of life and explore the city of Oslo. I would highly recommend GSERM to anyone seeking to develop their research methodology skills.
"
Somendra Narayan, PhD Strategic Management
Erasmus University Netherlands
"I had one of my most unique study experiences with GSERM Global School in Empirical Research Methods in Oslo. Classes at futuristic BI located 2km from foresty areas were short, but well-taught with top notch lecturers. Cross-country skiing and frosty sightseeing in Oslo gave me a glimps into the way of life in snowy, energetic and beautiful Norway. As a PhD student in natural sciences it was enriching to meet people from totally different fields and I did not expect to make so many new friends in such a short time."
Jonas Laukkonen Ravn, PhD Biology
Gent University, Belgium
"I gained very deep insights at the Experimental Design and Multivariate Data Analysis modules at GSERM St.Gallen 2016. GSERM was a perfect blend of research work and fun."
Segun Shogbanmu, PhD Student/ Research Associate
Lagos Business School
"GSERM Global School in Empirical Research Methods is a great place to learn, a great place to teach, and a great place to meet people from throughout Europe and elsewhere."
Prof. Andrew F. Hayes
University of Calgary, Haskayne School of Business
"Again, thank you for the opportunity to teach at the summer school. The students were excellent, as were your arrangements."
Prof. John Kruschke
Indiana University
"Thanks for organizing everything so well. I had a great time!"
Prof. Gerald Häubl
University of Alberta